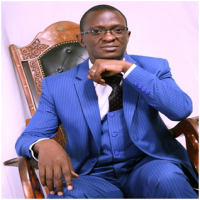
Peter Oluoch is the Creative Director, Doxa Interactive Studio, www.doxa.co.ke, a creative digital agency that specializes in Website Design, Video and Photography, Stocks Trading and Prediction, Data Science and Visualization and Books Writing.
Project Summary
Project Title: Prediction of Stock Prices Using Predictive Data Analytics: A Case of Nairobi Securities Exchange
Abstract: The field of stock trading worldwide is not even, has never been even and will not be even in the years to come. The money bags, the big boys continue to benefit and make massive profits from the stock market and the small fish continue to lose millions and billions of money in the market.
Thanks to technology and the fourth industrial revolution, thanks to big data, predictive analytics and more so Holt Winters Exponential Smoothening, it is now very possible to predict the volatility at the stock market and to make significant gains like the big boys. With the predictive analytics, the massive stocks data from the stock market becomes a goldmine that generates leads for short term investors who are able to be warned in advance of the possible capital erosion due to their bad investment stakes at the market and be warned of the possibility of capital gains before investing in the stock market. The data used in this project is a ten years’ data and hence the predictions is 90% accurate. While it is impossible to predict with 100% accuracy what will happen in the future since stock volatility is affected by many other economic, political, environmental factors, it is very much possible to use historical data from the Nairobi Securities Exchange, do stocks prediction and predict with accuracy the future stocks movement if all other factors that affect stock volatility are held constant. The constant, is one of the major assumptions in this project.
Unlike in the olden times where stock traders and stock market analysts would rely on fundamentals of a given company to decide whether to buy or sell, or rely on technical analysis by following graphs and trends, predicting the stock market is getting easier using predictive analytics. In the past, predicting the stock market volatility has been done by several technologies including Artificial Neural Networks, Decision Trees, Logic Regression, Ensemble Models, Bayesian Models among many other statistical models. This project looks specifically at Holt Winters Exponential Smoothing, a branch of supervised machine learning model.